We develop integrated predictive tools for operation and maintenance of machinery, fleet and processes. These tools guide operators to make corrective action proactively to prevent unnecessary shutdowns.
Key facts: smart predictive maintenance
Operation and maintenance data-analytics services for decision support
Identifying critical circumstances and notifying about the impending anomalies
Combining tribological root interactions, knowledge and data-driven analytics and hybrid modelling into predictive maintenance
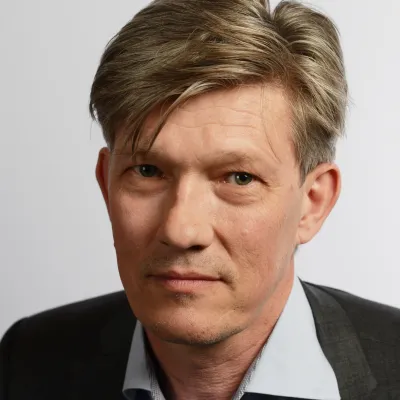