Digitalisation and sustainability are the two mega-trends in the mobility domain. Green transport endorses the use of alternative fuels and electric vehicles. The automotive and machinery industries have recently launched even heavy goods vehicles for the urban environment where the powertrain is electric. The second major mega-trend is the digitalisation of mobility at all levels, starting from a single vehicle and ending with fleet management. Operations that traditionally have been done manually are now not only recorded digitally but also processed to a suitable format automatically. Recent examples (ChatGPT [2], fotor [3], etc.) have been entering the markets and they have shown exceptional capabilities to use the existing data in the internet and generate graphs and reports etc. However, AI is also used in background data processing automatically, even though people are not even aware of the different AI layers.
AI history examples
Artificial intelligence took its first steps in the automotive industry around 2007 when the first DARPA urban challenge competitions were held in California. Of course, a certain type of intelligence has been a part of the automotive industry ever since the first sensors and CPUs were introduced in the mid-70s. However, this was still far from when the first neural networks were piloted in industrial applications (product quality checking, etc.) in the early 90’s even though the artificial neural networks (ANN) were first introduced in 1943 [1]. Due to the lack of computation power, it took more than 35 years before the first commercial applications came onto the markets. Interestingly, the reason for introducing neural-based computing has remained the same over the years: the need to process a huge amount of data.
In addition to physical road infrastructure, the digital road infrastructure started to become a hot topic when the smart traffic lights and especially the communication between vehicles and infrastructure were introduced from 2005 – 2012. The aim is to collect images not only from road cameras but also from infrastructure cameras and share the data in a standardised format between road users and authorities. In 2015, the research community was even talking about a physical internet where parcels are divided into small pieces and routes are optimised like the TCP/IP packages in the internet. Package delivery and route planning is one of the most traditional tasks for artificial intelligence due to the huge number of options and parameters to be optimised.
This data economy is one of the components and it motivates the use of more intelligent algorithms to convert data into a valuable format. Therefore, Google and many other companies have introduced map-based services for the mobility domain. Consequently, the companies and authorities that own the data have woken up to consider that the data is the oil of smart traffic solutions and therefore, it has value. Tesla is one of the pioneers in data processing from their vehicle fleet. They have the idea to 1) collect data from the vehicle, 2) process the data into a more valuable format and 3) sell the processed data back to the vehicle owner.
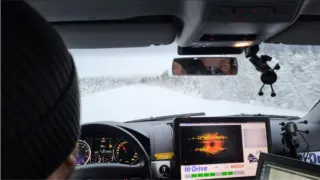
Navigation systems were introduced for a wider audience around the middle of the 90's when the first handheld devices were coming onto the mass markets. The first navigators did not use AI but soon when the maps became more versatile, they included various attributes to be updated continuously. There were manual updating centres where people and map providers sent updating requests for manual processing. Soon, it started to be considered if massive amounts of updates could be automated and if route planning could enclose not only the shortest route but also take into account fuel consumption and congestions in the route. The only sensible way was to implement artificial intelligence for optimising non-linear optimisation problems.
The major expected trends caused by penetration AI based systems to the markets:
Artificial intelligence requires high computational power:
- The automotive industry is partnering with chip providers to have computers on board (e.g., the co-development of Mercedes-Nvidia in Europe).
- Public transport operators are investing in both their own and cloud-based servers to process data more or less in real-time. Otherwise, the data pipelines may get stuck.
- Big players (like Google, IBM) are really looking at the opportunities to use quantum computing techniques for dedicated purposes in the background.
- A negative by-product is that server halls and CPUs are already today causing about 2% of the world's carbon dioxide emissions, and due to larger server rooms, this is expected to rise to 5-6%.
Capabilities to predict potential challenges from the data:
- The large amounts of data enable the utilization of mobility data to predict congestions and provide detours in advance. Today, the prediction is done for a couple of hours ahead, but in the future, travel times will be more accurate even weeks before.
- There are increasingly intelligent route planners for public transport where combinations of trams, buses, and shuttle service are merged with automated robot taxis, enabling real door-to-door transport.
Distributed AI system:
- The AI is localised to respect privacy rules and provide local services. The distributed intelligence has hierarchic nature : single hand-held device <=> vehicle <=> edge computing unit <=> cloud based computation. Single device is personalised to user behaviour whereas cloud based system has generalised features
- Distribution provides an opportunity for mobility stakeholders to provide local and personalised services (including safety warnings).
Privacy rules:
- It's becoming mandatory that also AI based solutions follow the privacy rules regulated by the European Commission. This prevents some of the applications to collect personal data including vehicle register plates. On the other hand, protection of personal data also limits personalising the services according to user needs. There is also danger that the U.S. companies will dominate the AI data markets and Europe is over-regulating AI approval.
Benefits of AI in automated driving
Automated driving functions
SAE introduced the definition for different automation levels starting from 0 (no automation) and ending at 5 (full automation everywhere). Still, an automation level up to 3 (partial automation) is called more of a driver assistance system instead of real automation levels. It's also slightly confusing what automation level 3 and 4 really mean, but nevertheless, 4 requires sophisticated systems where the computer dominates and the "driver" has only a rare assistance role. When considering highly automated driving, the computers in the vehicle need to process 1 Gigabyte of data in a second which is not possible by using deterministic algorithms and therefore, levels 4 and 5 are not possible without artificial intelligence recognising objects and taking care of vehicle decision making.
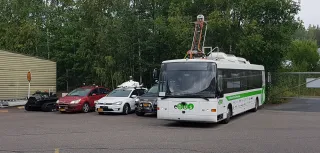
Sensor data
Modern vehicles are equipped with ADAS (emergency braking, lane keeping, etc.) which are based on camera and radar technologies. The algorithms are aimed at identifying objects in front, utilising information about speed, material properties, and extracting specific features like lane markings or traffic signs. These are based on the assumption that a metallic object detected by radar is a front vehicle or other obstacle like a fence which should be avoided. This works fairly well as long as the driver is in charge of final decision making and automation functions have only an assistance role.
Automated driving functions require more intelligence and much more data, not only for detecting objects in front but also to recognise the object. Such an example is a metallic can in front of the vehicle when hard braking is more dangerous for passengers than letting the vehicle drive through.
Trajectory and path planning
Selecting the right trajectory is an amazingly challenging problem. Typically, one overtaking on a motorway produces 5000 – 50000 different optional route alternatives. This depends on available free space, speed, accelerations, and distance to the vehicle in the rear. The problem is even more challenging if taken into account non-visible lane markings for example due to snow or having gravel roads. Passenger cars are usually operating in the areas where there are special tracks, curbs or lane markings available. However, this is not the case for example with the machineries which are operating in forest or other open/closed areas. Selecting an optimal route depends on many parameters like big rocks, distance between trees and softness of the ground. This is an optimal case where artificial intelligence can have major benefits but only if the training data is properly selected. Usually, there are no deterministic one prevailing answer but rather alternative routes having pros. and cons.
C-ITS
C-ITS standards have been available already for 10 years. Europe expected already in 2008 that connected driving is going to be booming in the next 5 years. That never happened even though that C-ITS has been deployed step by step but very slowly covering today backend public transport and some road maintenance services.
Artificial intelligence could be the key enabling technology to gain implementation of C-ITS services. Now the biggest issue is that there are information providers and users but there are very few stakeholders which convert the information to valuable format which users are willing to pay for. Taking an example – there are route planners to provide estimated time of arrival in different transport modalities and there are road weather services available. However, nobody estimates what the estimated impact of dense fog to different transport modes is.
Mobility services
Behaviour of people has already significantly changed in the urban areas. Public transport is becoming more and more popular due to restrictions on using and parking private cars in cities. However, public transport is not about big buses and metros like 15 years ago, but today, they also include scooters, shared cars, bikes, Uber, etc. One of the challenges is that cost and route optimisation changes depending on location and there are people who don’t want to use, for example, scooters.
Artificial intelligence has two aspects to provide better mobility services:
-
more personalized solutions according to favoured transport modalities and willingness to pay
-
expand the city type alternative to inter-urban areas where e.g. automated shuttle buses could serve people when transport demand is high (e.g. rush hours in railway stations or some specific events)
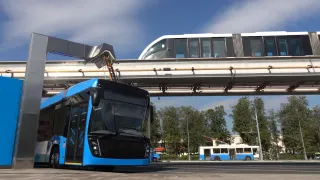
Conclusions
AI performance highly depends on the quality of training data. In the mobility domain, the other important aspect is the coverage of the training data. Real driving on roads is far from an ideal world. There are rare things like tyres lying on the highway which happens maybe once per year but may cause a serious, unacceptable accident. The online artificial intelligence frameworks (e.g., ChatGPT [2]) use the information available online. Some of the systems are using a cache to speed up the response time. Chatsonic [4] is an alternative to ChatGPT to process real online data. In the mobility context, the biggest benefit of ChatGPT is not probably the capability to produce reports but rather more interesting is the artificial intelligence for doing coding and software applications for mobility services.
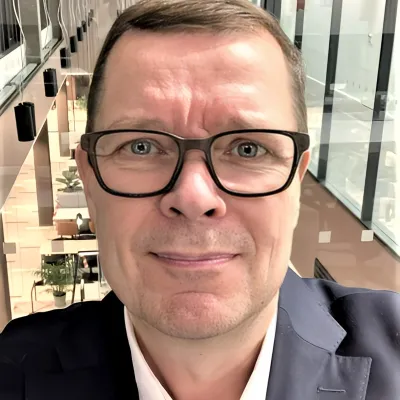